Accelerating ML Training And Delivery With In-Database Machine Learning
Data Engineering Podcast - Podcast autorstwa Tobias Macey - Niedziele
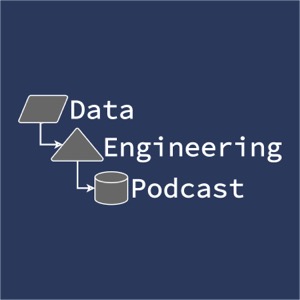
Kategorie:
Summary When you build a machine learning model, the first step is always to load your data. Typically this means downloading files from object storage, or querying a database. To speed up the process, why not build the model inside the database so that you don’t have to move the information? In this episode Paige Roberts explains the benefits of pushing the machine learning processing into the database layer and the approach that Vertica has taken for their implementation. If you are looking for a way to speed up your experimentation, or an easy way to apply AutoML then this conversation is for you. Announcements Hello and welcome to the Data Engineering Podcast, the show about modern data management When you’re ready to build your next pipeline, or want to test out the projects you hear about on the show, you’ll need somewhere to deploy it, so check out our friends at Linode. With their managed Kubernetes platform it’s now even easier to deploy and scale your workflows, or try out the latest Helm charts from tools like Pulsar and Pachyderm. With simple pricing, fast networking, object storage, and worldwide data centers, you’ve got everything you need to run a bulletproof data platform. Go to dataengineeringpodcast.com/linode today and get a $100 credit to try out a Kubernetes cluster of your own. And don’t forget to thank them for their continued support of this show! RudderStack’s smart customer data pipeline is warehouse-first. It builds your customer data warehouse and your identity graph on your data warehouse, with support for Snowflake, Google BigQuery, Amazon Redshift, and more. Their SDKs and plugins make event streaming easy, and their integrations with cloud applications like Salesforce and ZenDesk help you go beyond event streaming. With RudderStack you can use all of your customer data to answer more difficult questions and then send those insights to your whole customer data stack. Sign up free at dataengineeringpodcast.com/rudder today. We’ve all been asked to help with an ad-hoc request for data by the sales and marketing team. Then it becomes a critical report that they need updated every week or every day. Then what do you do? Send a CSV via email? Write some Python scripts to automate it? But what about incremental sync, API quotas, error handling, and all of the other details that eat up your time? Today, there is a better way. With Census, just write SQL or plug in your dbt models and start syncing your cloud warehouse to SaaS applications like Salesforce, Marketo, Hubspot, and many more. Go to dataengineeringpodcast.com/census today to get a free 14-day trial. Your host is Tobias Macey and today I’m interviewing Paige Roberts about machine learning workflows inside the database Interview Introduction How did you get involved in the area of data management? Can you start by giving an overview of the current state of the market for databases that support in-process machine learning? What are the motivating factors for running a machine learning workflow inside the database? What styles of ML are feasible to do inside the database? (e.g. bayesian inference, deep learning, etc.) What are the performance implications of running a model training pipeline within the database runtime? (both in terms of training performance boosts, and database performance impacts) Can you describe the architecture of how the machine learning process is managed by the database engine? How do you manage interacting with Python/R/Jupyter/etc. when working within the database? What is the impact on data pipeline and MLOps architectures when using the database to manage the machine learning workflow? What are the most interesting, innovative, or unexpected ways that you have seen in-database ML used? What are the most interesting, unexpected, or challenging lessons that you have learned while working on machine learning inside the database? When is in-database ML the wrong choice? What are the recent trends/changes in machine learning for the database that you are excited for? Contact Info LinkedIn Blog @RobertsPaige on Twitter @PaigeEwing on Twitter Parting Question From your perspective, what is the biggest gap in the tooling or technology for data management today? Closing Announcements Thank you for listening! Don’t forget to check out our other show, Podcast.__init__ to learn about the Python language, its community, and the innovative ways it is being used. Visit the site to subscribe to the show, sign up for the mailing list, and read the show notes. If you’ve learned something or tried out a project from the show then tell us about it! Email [email protected]) with your story. To help other people find the show please leave a review on iTunes and tell your friends and co-workers Join the community in the new Zulip chat workspace at dataengineeringpodcast.com/chat Links Vertica SyncSort Hortonworks Infoworld – 8 databases supporting in-database machine learning Power BI Podcast Episode Grafana Tableau K-Means Clustering MPP == Massively Parallel Processing AutoML Random Forest PMML == Predictive Model Markup Language SVM == Support Vector Machine Naive Bayes XGBoost Pytorch Tensorflow Neural Magic Tensorflow Frozen Graph Parquet ORC Avro CNCF == Cloud Native Computing Foundation Hotel California VerticaPy Pandas Podcast.__init__ Episode Jupyter Notebook UDX Unifying Analytics Presentation Hadoop Yarn Holden Karau Spark Vertica Academy The intro and outro music is from The Hug by The Freak Fandango Orchestra / CC BY-SA Support Data Engineering Podcast