Adding Support For Distributed Transactions To The Redpanda Streaming Engine
Data Engineering Podcast - Podcast autorstwa Tobias Macey - Niedziele
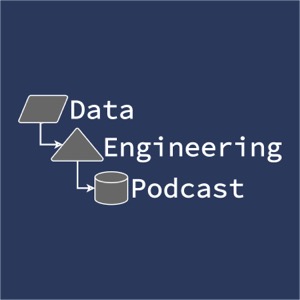
Kategorie:
Summary Transactions are a necessary feature for ensuring that a set of actions are all performed as a single unit of work. In streaming systems this is necessary to ensure that a set of messages or transformations are all executed together across different queues. In this episode Denis Rystsov explains how he added support for transactions to the Redpanda streaming engine. He discusses the use cases for transactions, the different strategies, semantics, and guarantees that they might need to support, and how his implementation ended up improving the performance of bulk write operations. This is an interesting deep dive into the internals of a high performance streaming engine and the details that are involved in building distributed systems. Announcements Hello and welcome to the Data Engineering Podcast, the show about modern data management When you’re ready to build your next pipeline, or want to test out the projects you hear about on the show, you’ll need somewhere to deploy it, so check out our friends at Linode. With their managed Kubernetes platform it’s now even easier to deploy and scale your workflows, or try out the latest Helm charts from tools like Pulsar and Pachyderm. With simple pricing, fast networking, object storage, and worldwide data centers, you’ve got everything you need to run a bulletproof data platform. Go to dataengineeringpodcast.com/linode today and get a $100 credit to try out a Kubernetes cluster of your own. And don’t forget to thank them for their continued support of this show! Struggling with broken pipelines? Stale dashboards? Missing data? If this resonates with you, you’re not alone. Data engineers struggling with unreliable data need look no further than Monte Carlo, the world’s first end-to-end, fully automated Data Observability Platform! In the same way that application performance monitoring ensures reliable software and keeps application downtime at bay, Monte Carlo solves the costly problem of broken data pipelines. Monte Carlo monitors and alerts for data issues across your data warehouses, data lakes, ETL, and business intelligence, reducing time to detection and resolution from weeks or days to just minutes. Start trusting your data with Monte Carlo today! Visit dataengineeringpodcast.com/impact today to save your spot at IMPACT: The Data Observability Summit a half-day virtual event featuring the first U.S. Chief Data Scientist, founder of the Data Mesh, Creator of Apache Airflow, and more data pioneers spearheading some of the biggest movements in data. The first 50 to RSVP with this link will be entered to win an Oculus Quest 2 — Advanced All-In-One Virtual Reality Headset. RSVP today – you don’t want to miss it! Atlan is a collaborative workspace for data-driven teams, like Github for engineering or Figma for design teams. By acting as a virtual hub for data assets ranging from tables and dashboards to SQL snippets & code, Atlan enables teams to create a single source of truth for all their data assets, and collaborate across the modern data stack through deep integrations with tools like Snowflake, Slack, Looker and more. Go to dataengineeringpodcast.com/atlan today and sign up for a free trial. If you’re a data engineering podcast listener, you get credits worth $3000 on an annual subscription Your host is Tobias Macey and today I’m interviewing Denis Rystsov about implementing transactions in the RedPanda streaming engine Interview Introduction How did you get involved in the area of data management? Can you quickly recap what RedPanda is and the goals of the project? What are the use cases for transactions in a pub/sub messaging system? What are the elements of streaming systems that make atomic transactions a complex problem? What was the motivation for starting down the path of adding transactions to the RedPanda engine? How did the constraint of supporting the Kafka API influence your implementation strategy for transaction semantics? Can you talk through the details of how you ended up implementing transactions in RedPanda? What are some of the roadblocks and complexities that you encountered while working through the implementation? How did you approach the validation and verification of the transactions? What other features or capabilities are you planning to work on next? What are the most interesting, innovative, or unexpected ways that you have seen transactions in RedPanda used? What are the most interesting, unexpected, or challenging lessons that you have learned while working on transactions for RedPanda? When are transactions the wrong choice? What do you have planned for the future of transaction support in RedPanda? Contact Info @rystsov on twitter LinkedIn Parting Question From your perspective, what is the biggest gap in the tooling or technology for data management today? Links Vectorized RedPanda Podcast Episode RedPanda Transactions Post Yandex Cassandra MongoDB Riak Cosmos DB Jepsen Podcast Episode Testing Shared Memories paper Journal of Systems Research Kafka Pulsar Seastar Framework CockroachDB Podcast Episode TiDB Calvin Paper Polyjuice Paper Parallel Commit Chaos Testing Matchmaker Paxos Algorithm The intro and outro music is from The Hug by The Freak Fandango Orchestra / CC BY-SA Support Data Engineering Podcast