Building A Cost Effective Data Catalog With Tree Schema
Data Engineering Podcast - Podcast autorstwa Tobias Macey - Niedziele
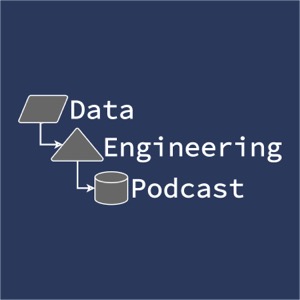
Kategorie:
Summary A data catalog is a critical piece of infrastructure for any organization who wants to build analytics products, whether internal or external. While there are a number of platforms available for building that catalog, many of them are either difficult to deploy and integrate, or expensive to use at scale. In this episode Grant Seward explains how he built Tree Schema to be an easy to use and cost effective option for organizations to build their data catalogs. He also shares the internal architecture, how he approached the design to make it accessible and easy to use, and how it autodiscovers the schemas and metadata for your source systems. Announcements Hello and welcome to the Data Engineering Podcast, the show about modern data management What are the pieces of advice that you wish you had received early in your career of data engineering? If you hand a book to a new data engineer, what wisdom would you add to it? I’m working with O’Reilly on a project to collect the 97 things that every data engineer should know, and I need your help. Go to dataengineeringpodcast.com/97things to add your voice and share your hard-earned expertise. When you’re ready to build your next pipeline, or want to test out the projects you hear about on the show, you’ll need somewhere to deploy it, so check out our friends at Linode. With their managed Kubernetes platform it’s now even easier to deploy and scale your workflows, or try out the latest Helm charts from tools like Pulsar and Pachyderm. With simple pricing, fast networking, object storage, and worldwide data centers, you’ve got everything you need to run a bulletproof data platform. Go to dataengineeringpodcast.com/linode today and get a $60 credit to try out a Kubernetes cluster of your own. And don’t forget to thank them for their continued support of this show! Modern Data teams are dealing with a lot of complexity in their data pipelines and analytical code. Monitoring data quality, tracing incidents, and testing changes can be daunting and often takes hours to days. Datafold helps Data teams gain visibility and confidence in the quality of their analytical data through data profiling, column-level lineage and intelligent anomaly detection. Datafold also helps automate regression testing of ETL code with its Data Diff feature that instantly shows how a change in ETL or BI code affects the produced data, both on a statistical level and down to individual rows and values. Datafold integrates with all major data warehouses as well as frameworks such as Airflow & dbt and seamlessly plugs into CI workflows. Follow go.datafold.com/dataengineeringpodcast to start a 30-day trial of Datafold. Once you sign up and create an alert in Datafold for your company data, they will send you a cool water flask. Are you bogged down by having to manually manage data access controls, repeatedly move and copy data, and create audit reports to prove compliance? How much time could you save if those tasks were automated across your cloud platforms? Immuta is an automated data governance solution that enables safe and easy data analytics in the cloud. Our comprehensive data-level security, auditing and de-identification features eliminate the need for time-consuming manual processes and our focus on data and compliance team collaboration empowers you to deliver quick and valuable data analytics on the most sensitive data to unlock the full potential of your cloud data platforms. Learn how we streamline and accelerate manual processes to help you derive real results from your data at dataengineeringpodcast.com/immuta. Your host is Tobias Macey and today I’m interviewing Grant Seward about Tree Schema, a human friendly data catalog Interview Introduction How did you get involved in the area of data management? Can you start by giving an overview of what you have built at Tree Schema? What was your motivation for creating it? At what stage of maturity should a team or organization consider a data catalog to be a necessary component in their data platform? There are a large and growing number of projects and products designed to provide a data catalog, with each of them addressing the problem in a slightly different way. What are the necessary elements for a data catalog? How does Tree Schema compare to the available options? (e.g. Amundsen, Company Wiki, Metacat, Metamapper, etc.) How is the Tree Schema system implemented? How has the design or direction of Tree Schema evolved since you first began working on it? How did you approach the schema definitions for defining entities? What was your guiding heuristic for determining how to design the interface and data models? – I wrote down notes that combine this with the question above How do you handle integrating with data sources? In addition to storing schema information you allow users to store information about the transformations being performed. How is that represented? How can users populate information about their transformations in an automated fashion? How do you approach evolution and versioning of schema information? What are the scaling limitations of tree schema, whether in terms of the technical or cognitive complexity that it can handle? What are some of the most interesting, innovative, or unexpected ways that you have seen Tree Schema being used? What have you found to be the most interesting, unexpected, or challenging lessons learned in the process of building and promoting Tree Schema? When is Tree Schema the wrong choice? What do you have planned for the future of the product? Contact Info Email Linkedin Parting Question From your perspective, what is the biggest gap in the tooling or technology for data management today? Closing Announcements Thank you for listening! Don’t forget to check out our other show, Podcast.__init__ to learn about the Python language, its community, and the innovative ways it is being used. Visit the site to subscribe to the show, sign up for the mailing list, and read the show notes. If you’ve learned something or tried out a project from the show then tell us about it! Email [email protected]) with your story. To help other people find the show please leave a review on iTunes and tell your friends and co-workers Join the community in the new Zulip chat workspace at dataengineeringpodcast.com/chat Links Tree Schema Tree Schema – Data Lineage as Code Capital One Walmart Labs Data Catalog Data Discovery Amundsen Metacat Marquez Metamapper Infoworks Collibra Faust Podcast.__init__ Episode Django PostgreSQL Redis Celery Amazon ECS (Elastic Container Service) Django Storages Dagster Airflow DataHub Avro Singer Apache Atlas The intro and outro music is from The Hug by The Freak Fandango Orchestra / CC BY-SA Support Data Engineering Podcast