Optimize Your Machine Learning Development And Serving With The Open Source Vector Database Milvus
Data Engineering Podcast - Podcast autorstwa Tobias Macey - Niedziele
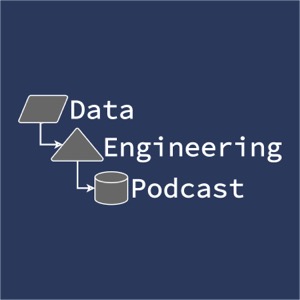
Kategorie:
Summary The optimal format for storage and retrieval of data is dependent on how it is going to be used. For analytical systems there are decades of investment in data warehouses and various modeling techniques. For machine learning applications relational models require additional processing to be directly useful, which is why there has been a growth in the use of vector databases. These platforms store direct representations of the vector embeddings that machine learning models rely on for computing relevant predictions so that there is no additional processing required to go from input data to inference output. In this episode Frank Liu explains how the open source Milvus vector database is implemented to speed up machine learning development cycles, how to think about proper storage and scaling of these vectors, and how data engineering and machine learning teams can collaborate on the creation and maintenance of these data sets. Announcements Hello and welcome to the Data Engineering Podcast, the show about modern data management When you’re ready to build your next pipeline, or want to test out the projects you hear about on the show, you’ll need somewhere to deploy it, so check out our friends at Linode. With their new managed database service you can launch a production ready MySQL, Postgres, or MongoDB cluster in minutes, with automated backups, 40 Gbps connections from your application hosts, and high throughput SSDs. Go to dataengineeringpodcast.com/linode today and get a $100 credit to launch a database, create a Kubernetes cluster, or take advantage of all of their other services. And don’t forget to thank them for their continued support of this show! Data stacks are becoming more and more complex. This brings infinite possibilities for data pipelines to break and a host of other issues, severely deteriorating the quality of the data and causing teams to lose trust. Sifflet solves this problem by acting as an overseeing layer to the data stack – observing data and ensuring it’s reliable from ingestion all the way to consumption. Whether the data is in transit or at rest, Sifflet can detect data quality anomalies, assess business impact, identify the root cause, and alert data teams’ on their preferred channels. All thanks to 50+ quality checks, extensive column-level lineage, and 20+ connectors across the Data Stack. In addition, data discovery is made easy through Sifflet’s information-rich data catalog with a powerful search engine and real-time health statuses. Listeners of the podcast will get $2000 to use as platform credits when signing up to use Sifflet. Sifflet also offers a 2-week free trial. Find out more at dataengineeringpodcast.com/sifflet today! RudderStack helps you build a customer data platform on your warehouse or data lake. Instead of trapping data in a black box, they enable you to easily collect customer data from the entire stack and build an identity graph on your warehouse, giving you full visibility and control. Their SDKs make event streaming from any app or website easy, and their state-of-the-art reverse ETL pipelines enable you to send enriched data to any cloud tool. Sign up free… or just get the free t-shirt for being a listener of the Data Engineering Podcast at dataengineeringpodcast.com/rudder. Data teams are increasingly under pressure to deliver. According to a recent survey by Ascend.io, 95% in fact reported being at or over capacity. With 72% of data experts reporting demands on their team going up faster than they can hire, it’s no surprise they are increasingly turning to automation. In fact, while only 3.5% report having current investments in automation, 85% of data teams plan on investing in automation in the next 12 months. 85%!!! That’s where our friends at Ascend.io come in. The Ascend Data Automation Cloud provides a unified platform for data ingestion, transformation, orchestration, and observability. Ascend users love its declarative pipelines, powerful SDK, elegant UI, and extensible plug-in architecture, as well as its support for Python, SQL, Scala, and Java. Ascend automates workloads on Snowflake, Databricks, BigQuery, and open source Spark, and can be deployed in AWS, Azure, or GCP. Go to dataengineeringpodcast.com/ascend and sign up for a free trial. If you’re a data engineering podcast listener, you get credits worth $5,000 when you become a customer. Your host is Tobias Macey and today I’m interviewing Frank Liu about the open source vector database Milvus and how it simplifies the work of supporting ML teams Interview Introduction How did you get involved in the area of data management? Can you describe what Milvus is and the story behind it? What are the goals of the project? Who is the target audience for this database? What are the use cases for a vector database and similarity search of vector embeddings? What are some of the unique capabilities that this category of database engine introduces? Can you describe how Milvus is architected? What are the primary system requirements that have influenced the design choices? How have the goals and implementation evolved since you started working on it? What are some of the interesting details that you have had to address in the storage layer to allow for fast and efficient retrieval of vector embeddings? What are the limitations that you have had to impose on size or dimensionality of vectors to allow for a consistent user experience in a running system? The reference material states that similarity between two vectors implies similarity in the source data. What are some of the characteristics of vector embeddings that might make them immune or susceptible to confusion of similarity across different source data types that share some implicit relationship due to specifics of their vectorized representation? (e.g. an image vs. an audio file, etc.) What are the available deployment models/targets and how does that influence potential use cases? What is the workflow for someone who is building an application on top of Milvus? What are some of the data management considerations that are introduced by vector databases? (e.g. manage versions of vectors, metadata management, etc.) What are the most interesting, innovative, or unexpected ways that you have seen Milvus used? What are the most interesting, unexpected, or challenging lessons that you have learned while working on Milvus? When is Milvus the wrong choice? What do you have planned for the future of Milvus? Contact Info LinkedIn fzliu on GitHub Website Parting Question From your perspective, what is the biggest gap in the tooling or technology for data management today? Closing Announcements Thank you for listening! Don’t forget to check out our other shows. Podcast.__init__ covers the Python language, its community, and the innovative ways it is being used. The Machine Learning Podcast helps you go from idea to production with machine learning. Visit the site to subscribe to the show, sign up for the mailing list, and read the show notes. If you’ve learned something or tried out a project from the show then tell us about it! Email [email protected]) with your story. To help other people find the show please leave a review on Apple Podcasts and tell your friends and co-workers Links Milvus Zilliz Linux Foundation/AI & Data MySQL PostgreSQL CockroachDB Pilosa Podcast Episode Pinecone Vector DB Podcast Episode Vector Embedding Reverse Image Search Vector Arithmetic Vector Distance SIGMOD Tensor Rotation Matrix L2 Distance Cosine Distance OpenAI CLIP Knowhere Kafka Pulsar Podcast Episode CAP Theorem Milvus Helm Chart Zilliz Cloud MinIO Towhee Attu Feder FPGA == Field Programmable Gate Array TPU == Tensor Processing Unit The intro and outro music is from The Hug by The Freak Fandango Orchestra / CC BY-SA Support Data Engineering Podcast