Repeatable Patterns For Designing Data Platforms And When To Customize Them
Data Engineering Podcast - Podcast autorstwa Tobias Macey - Niedziele
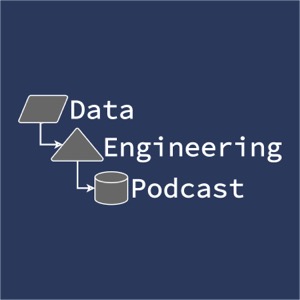
Kategorie:
Summary Building a data platform for your organization is a challenging undertaking. Building multiple data platforms for other organizations as a service without burning out is another thing entirely. In this episode Brandon Beidel from Red Ventures shares his experiences as a data product manager in charge of helping his customers build scalable analytics systems that fit their needs. He explains the common patterns that have been useful across multiple use cases, as well as when and how to build customized solutions. Announcements Hello and welcome to the Data Engineering Podcast, the show about modern data management When you’re ready to build your next pipeline, or want to test out the projects you hear about on the show, you’ll need somewhere to deploy it, so check out our friends at Linode. With their managed Kubernetes platform it’s now even easier to deploy and scale your workflows, or try out the latest Helm charts from tools like Pulsar and Pachyderm. With simple pricing, fast networking, object storage, and worldwide data centers, you’ve got everything you need to run a bulletproof data platform. Go to dataengineeringpodcast.com/linode today and get a $100 credit to try out a Kubernetes cluster of your own. And don’t forget to thank them for their continued support of this show! This episode is brought to you by Acryl Data, the company behind DataHub, the leading developer-friendly data catalog for the modern data stack. Open Source DataHub is running in production at several companies like Peloton, Optum, Udemy, Zynga and others. Acryl Data provides DataHub as an easy to consume SaaS product which has been adopted by several companies. Signup for the SaaS product at dataengineeringpodcast.com/acryl Hey Data Engineering Podcast listeners, want to learn how the Joybird data team reduced their time spent building new integrations and managing data pipelines by 93%? Join our live webinar on April 20th. Joybird director of analytics, Brett Trani, will walk through how retooling their data stack with RudderStack, Snowflake, and Iterable made this possible. Visit www.rudderstack.com/joybird?utm_source=rss&utm_medium=rss to register today. The most important piece of any data project is the data itself, which is why it is critical that your data source is high quality. PostHog is your all-in-one product analytics suite including product analysis, user funnels, feature flags, experimentation, and it’s open source so you can host it yourself or let them do it for you! You have full control over your data and their plugin system lets you integrate with all of your other data tools, including data warehouses and SaaS platforms. Give it a try today with their generous free tier at dataengineeringpodcast.com/posthog Your host is Tobias Macey and today I’m interviewing Brandon Beidel about his data platform journey at Red Ventures Interview Introduction How did you get involved in the area of data management? Can you describe what Red Ventures is and your role there? Given the relative newness of data product management, where do you draw inspiration and direction for how to approach your work? What are the primary categories of data product that your data consumers are building/relying on? What are the types of data sources that you are working with to power those downstream use cases? Can you describe the size and composition/organization of your data team(s)? How do you approach the build vs. buy decision while designing and evolving your data platform? What are the tools/platforms/architectural and usage patterns that you and your team have developed for your platform? What are the primary goals and constraints that have contributed to your decisions? How have the goals and design of the platform changed or evolved since you started working with the team? You recently went through the process of establishing and reporting on SLAs for your data products. Can you describe the approach you took and the useful lessons that were learned? What are the technical and organizational components of the data work at Red Ventures that have proven most difficult? What excites you most about the future of data engineering? What are the most interesting, innovative, or unexpected ways that you have seen teams building more reliable data systems? What aspects of data tooling or processes are still missing for most data teams? What are the most interesting, unexpected, or challenging lessons that you have learned while working on data products at Red Ventures? What do you have planned for the future of your data platform? Contact Info LinkedIn Parting Question From your perspective, what is the biggest gap in the tooling or technology for data management today? Closing Announcements Thank you for listening! Don’t forget to check out our other show, Podcast.__init__ to learn about the Python language, its community, and the innovative ways it is being used. Visit the site to subscribe to the show, sign up for the mailing list, and read the show notes. If you’ve learned something or tried out a project from the show then tell us about it! Email [email protected]) with your story. To help other people find the show please leave a review on iTunes and tell your friends and co-workers Links Red Ventures Monte Carlo Opportunity Cost dbt Podcast Episode Apache Ranger Privacera Podcast Episode Segment Fivetran Podcast Episode Databricks Bigquery Redshift Hightouch Podcast Episode Airflow Astronomer Podcast Episode Airbyte Podcast Episode Clickhouse Podcast Episode Presto Podcast Episode Trino The intro and outro music is from The Hug by The Freak Fandango Orchestra / CC BY-SA Support Data Engineering Podcast