Scalable Strategies For Protecting Data Privacy In Your Shared Data Sets
Data Engineering Podcast - Podcast autorstwa Tobias Macey - Niedziele
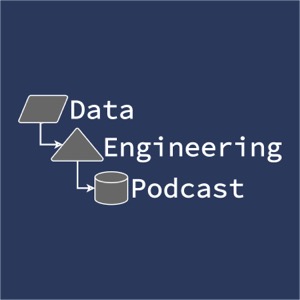
Kategorie:
Summary There are many dimensions to the work of protecting the privacy of users in our data. When you need to share a data set with other teams, departments, or businesses then it is of utmost importance that you eliminate or obfuscate personal information. In this episode Will Thompson explores the many ways that sensitive data can be leaked, re-identified, or otherwise be at risk, as well as the different strategies that can be employed to mitigate those attack vectors. He also explains how he and his team at Privacy Dynamics are working to make those strategies more accessible to organizations so that you can focus on all of the other tasks required of you. Announcements Hello and welcome to the Data Engineering Podcast, the show about modern data management When you’re ready to build your next pipeline, or want to test out the projects you hear about on the show, you’ll need somewhere to deploy it, so check out our friends at Linode. With their managed Kubernetes platform it’s now even easier to deploy and scale your workflows, or try out the latest Helm charts from tools like Pulsar and Pachyderm. With simple pricing, fast networking, object storage, and worldwide data centers, you’ve got everything you need to run a bulletproof data platform. Go to dataengineeringpodcast.com/linode today and get a $100 credit to try out a Kubernetes cluster of your own. And don’t forget to thank them for their continued support of this show! Today’s episode is Sponsored by Prophecy.io – the low-code data engineering platform for the cloud. Prophecy provides an easy-to-use visual interface to design & deploy data pipelines on Apache Spark & Apache Airflow. Now all the data users can use software engineering best practices – git, tests and continuous deployment with a simple to use visual designer. How does it work? – You visually design the pipelines, and Prophecy generates clean Spark code with tests on git; then you visually schedule these pipelines on Airflow. You can observe your pipelines with built in metadata search and column level lineage. Finally, if you have existing workflows in AbInitio, Informatica or other ETL formats that you want to move to the cloud, you can import them automatically into Prophecy making them run productively on Spark. Create your free account today at dataengineeringpodcast.com/prophecy. The only thing worse than having bad data is not knowing that you have it. With Bigeye’s data observability platform, if there is an issue with your data or data pipelines you’ll know right away and can get it fixed before the business is impacted. Bigeye let’s data teams measure, improve, and communicate the quality of your data to company stakeholders. With complete API access, a user-friendly interface, and automated yet flexible alerting, you’ve got everything you need to establish and maintain trust in your data. Go to dataengineeringpodcast.com/bigeye today to sign up and start trusting your analyses. Your host is Tobias Macey and today I’m interviewing Will Thompson about managing data privacy concerns for data sets used in analytics and machine learning Interview Introduction How did you get involved in the area of data management? Data privacy is a multi-faceted problem domain. Can you start by enumerating the different categories of privacy concern that are involved in analytical use cases? Can you describe what Privacy Dynamics is and the story behind it? Which categor(y|ies) are you focused on addressing? What are some of the best practices in the definition, protection, and enforcement of data privacy policies? Is there a data security/privacy equivalent to the OWASP top 10? What are some of the techniques that are available for anonymizing data while maintaining statistical utility/significance? What are some of the engineering/systems capabilities that are required for data (platform) engineers to incorporate these practices in their platforms? What are the tradeoffs of encryption vs. obfuscation when anonymizing data? What are some of the types of PII that are non-obvious? What are the risks associated with data re-identification, and what are some of the vectors that might be exploited to achieve that? How can privacy risks mitigation be maintained as new data sources are introduced that might contribute to these re-identification vectors? Can you describe how Privacy Dynamics is implemented? What are the most challenging engineering problems that you are dealing with? How do you approach validation of a data set’s privacy? What have you found to be useful heuristics for identifying private data? What are the risks of false positives vs. false negatives? Can you describe what is involved in integrating the Privacy Dynamics system into an existing data platform/warehouse? What would be required to integrate with systems such as Presto, Clickhouse, Druid, etc.? What are the most interesting, innovative, or unexpected ways that you have seen Privacy Dynamics used? What are the most interesting, unexpected, or challenging lessons that you have learned while working on Privacy Dynamics? When is Privacy Dynamics the wrong choice? What do you have planned for the future of Privacy Dynamics? Contact Info LinkedIn @willseth on Twitter Parting Question From your perspective, what is the biggest gap in the tooling or technology for data management today? Closing Announcements Thank you for listening! Don’t forget to check out our other show, Podcast.__init__ to learn about the Python language, its community, and the innovative ways it is being used. Visit the site to subscribe to the show, sign up for the mailing list, and read the show notes. If you’ve learned something or tried out a project from the show then tell us about it! Email [email protected]) with your story. To help other people find the show please leave a review on iTunes and tell your friends and co-workers Links Privacy Dynamics Pandas Podcast Episode – Pandas For Data Engineering Homomorphic Encryption Differential Privacy Immuta Podcast Episode The intro and outro music is from The Hug by The Freak Fandango Orchestra / CC BY-SA Support Data Engineering Podcast