Synthetic Data As A Service For Simplifying Privacy Engineering With Gretel
Data Engineering Podcast - Podcast autorstwa Tobias Macey - Niedziele
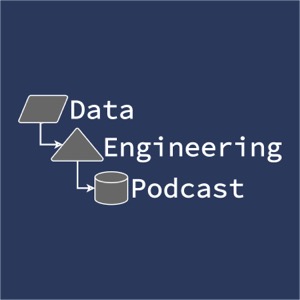
Kategorie:
Summary Any time that you are storing data about people there are a number of privacy and security considerations that come with it. Privacy engineering is a growing field in data management that focuses on how to protect attributes of personal data so that the containing datasets can be shared safely. In this episode Gretel co-founder and CTO John Myers explains how they are building tools for data engineers and analysts to incorporate privacy engineering techniques into their workflows and validate the safety of their data against re-identification attacks. Announcements Hello and welcome to the Data Engineering Podcast, the show about modern data management When you’re ready to build your next pipeline, or want to test out the projects you hear about on the show, you’ll need somewhere to deploy it, so check out our friends at Linode. With their managed Kubernetes platform it’s now even easier to deploy and scale your workflows, or try out the latest Helm charts from tools like Pulsar and Pachyderm. With simple pricing, fast networking, object storage, and worldwide data centers, you’ve got everything you need to run a bulletproof data platform. Go to dataengineeringpodcast.com/linode today and get a $100 credit to try out a Kubernetes cluster of your own. And don’t forget to thank them for their continued support of this show! This episode is brought to you by Acryl Data, the company behind DataHub, the leading developer-friendly data catalog for the modern data stack. Open Source DataHub is running in production at several companies like Peloton, Optum, Udemy, Zynga and others. Acryl Data provides DataHub as an easy to consume SaaS product which has been adopted by several companies. Signup for the SaaS product at dataengineeringpodcast.com/acryl Are you looking for a structured and battle-tested approach for learning data engineering? Would you like to know how you can build proper data infrastructures that are built to last? Would you like to have a seasoned industry expert guide you and answer all your questions? Join Pipeline Academy, the worlds first data engineering bootcamp. Learn in small groups with likeminded professionals for 9 weeks part-time to level up in your career. The course covers the most relevant and essential data and software engineering topics that enable you to start your journey as a professional data engineer or analytics engineer. Plus we have AMAs with world-class guest speakers every week! The next cohort starts in April 2022. Visit dataengineeringpodcast.com/academy and apply now! RudderStack helps you build a customer data platform on your warehouse or data lake. Instead of trapping data in a black box, they enable you to easily collect customer data from the entire stack and build an identity graph on your warehouse, giving you full visibility and control. Their SDKs make event streaming from any app or website easy, and their state-of-the-art reverse ETL pipelines enable you to send enriched data to any cloud tool. Sign up free… or just get the free t-shirt for being a listener of the Data Engineering Podcast at dataengineeringpodcast.com/rudder. Your host is Tobias Macey and today I’m interviewing John Myers about privacy engineering and use cases for synthetic data Interview Introduction How did you get involved in the area of data management? Can you describe what Gretel is and the story behind it? How do you define "privacy engineering"? In an organization or data team, who is typically responsible for privacy engineering? How would you characterize the current state of the art and adoption for privacy engineering? Who are the target users of Gretel and how does that inform the features and design of the product? What are the stages of the data lifecycle where Gretel is used? Can you describe a typical workflow for integrating Gretel into data pipelines for business analytics or ML model training? How is the Gretel platform implemented? How have the design and goals of the system changed or evolved since you started working on it? What are some of the nuances of synthetic data generation or masking that data engineers/data analysts need to be aware of as they start using Gretel? What are the most interesting, innovative, or unexpected ways that you have seen Gretel used? What are the most interesting, unexpected, or challenging lessons that you have learned while working on Gretel? When is Gretel the wrong choice? What do you have planned for the future of Gretel? Contact Info LinkedIn @jtm_tech on Twitter Parting Question From your perspective, what is the biggest gap in the tooling or technology for data management today? Closing Announcements Thank you for listening! Don’t forget to check out our other show, Podcast.__init__ to learn about the Python language, its community, and the innovative ways it is being used. Visit the site to subscribe to the show, sign up for the mailing list, and read the show notes. If you’ve learned something or tried out a project from the show then tell us about it! Email [email protected]) with your story. To help other people find the show please leave a review on iTunes and tell your friends and co-workers Links Gretel Privacy Engineering Weights and Biases Red Team/Blue Team Generative Adversarial Network Capture The Flag in application security CVE == Common Vulnerabilities and Exposures Machine Learning Cold Start Problem Faker Mockaroo Kaggle Sentry The intro and outro music is from The Hug by The Freak Fandango Orchestra / CC BY-SA Support Data Engineering Podcast