Using Product Driven Development To Improve The Productivity And Effectiveness Of Your Data Teams
Data Engineering Podcast - Podcast autorstwa Tobias Macey - Niedziele
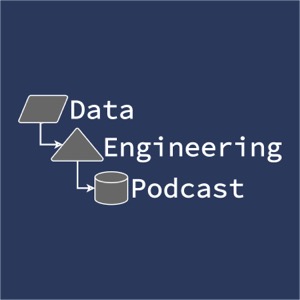
Kategorie:
Summary With all of the messaging about treating data as a product it is becoming difficult to know what that even means. Vishal Singh is the head of products at Starburst which means that he has to spend all of his time thinking and talking about the details of product thinking and its application to data. In this episode he shares his thoughts on the strategic and tactical elements of moving your work as a data professional from being task-oriented to being product-oriented and the long term improvements in your productivity that it provides. Announcements Hello and welcome to the Data Engineering Podcast, the show about modern data management When you're ready to build your next pipeline, or want to test out the projects you hear about on the show, you'll need somewhere to deploy it, so check out our friends at Linode. With their new managed database service you can launch a production ready MySQL, Postgres, or MongoDB cluster in minutes, with automated backups, 40 Gbps connections from your application hosts, and high throughput SSDs. Go to dataengineeringpodcast.com/linode today and get a $100 credit to launch a database, create a Kubernetes cluster, or take advantage of all of their other services. And don't forget to thank them for their continued support of this show! Modern data teams are dealing with a lot of complexity in their data pipelines and analytical code. Monitoring data quality, tracing incidents, and testing changes can be daunting and often takes hours to days or even weeks. By the time errors have made their way into production, it’s often too late and damage is done. Datafold built automated regression testing to help data and analytics engineers deal with data quality in their pull requests. Datafold shows how a change in SQL code affects your data, both on a statistical level and down to individual rows and values before it gets merged to production. No more shipping and praying, you can now know exactly what will change in your database! Datafold integrates with all major data warehouses as well as frameworks such as Airflow & dbt and seamlessly plugs into CI workflows. Visit dataengineeringpodcast.com/datafold today to book a demo with Datafold. RudderStack helps you build a customer data platform on your warehouse or data lake. Instead of trapping data in a black box, they enable you to easily collect customer data from the entire stack and build an identity graph on your warehouse, giving you full visibility and control. Their SDKs make event streaming from any app or website easy, and their extensive library of integrations enable you to automatically send data to hundreds of downstream tools. Sign up free at dataengineeringpodcast.com/rudder Build Data Pipelines. Not DAGs. That’s the spirit behind Upsolver SQLake, a new self-service data pipeline platform that lets you build batch and streaming pipelines without falling into the black hole of DAG-based orchestration. All you do is write a query in SQL to declare your transformation, and SQLake will turn it into a continuous pipeline that scales to petabytes and delivers up to the minute fresh data. SQLake supports a broad set of transformations, including high-cardinality joins, aggregations, upserts and window operations. Output data can be streamed into a data lake for query engines like Presto, Trino or Spark SQL, a data warehouse like Snowflake or Redshift., or any other destination you choose. Pricing for SQLake is simple. You pay $99 per terabyte ingested into your data lake using SQLake, and run unlimited transformation pipelines for free. That way data engineers and data users can process to their heart’s content without worrying about their cloud bill. For data engineering podcast listeners, we’re offering a 30 day trial with unlimited data, so go to dataengineeringpodcast.com/upsolver today and see for yourself how to avoid DAG hell. Your host is Tobias Macey and today I'm interviewing Vishal Singh about his experience building data products at Starburst Interview Introduction How did you get involved in the area of data management? Can you describe what your definition of a "data product" is? What are some of the different contexts in which the idea of a data product is applicable? How do the parameters of a data product change across those different contexts/consumers? What are some of the ways that you see the conversation around the purpose and practice of building data products getting overloaded by conflicting objectives? What do you see as common challenges in data teams around how to approach product thinking in their day-to-day work? What are some of the tactical ways that product-oriented work on data problems differs from what has become common practice in data teams? What are some of the features that you are building at Starburst that contribute to the efforts of data teams to build full-featured product experiences for their data? What are the most interesting, innovative, or unexpected ways that you have seen Starburst used in the context of data products? What are the most interesting, unexpected, or challenging lessons that you have learned while working at Starburst? When is a data product the wrong choice? What do you have planned for the future of support for data product development at Starburst? Contact Info LinkedIn @vishal_singh on Twitter Parting Question From your perspective, what is the biggest gap in the tooling or technology for data management today? Closing Announcements Thank you for listening! Don't forget to check out our other shows. Podcast.__init__ covers the Python language, its community, and the innovative ways it is being used. The Machine Learning Podcast helps you go from idea to production with machine learning. Visit the site to subscribe to the show, sign up for the mailing list, and read the show notes. If you've learned something or tried out a project from the show then tell us about it! Email [email protected]) with your story. To help other people find the show please leave a review on Apple Podcasts and tell your friends and co-workers Links Starburst Podcast Episode Geophysics Product-Led Growth Trino DataNova Starburst Galaxy Tableau PowerBI Podcast Episode Metabase Podcast Episode Great Expectations Podcast Episode The intro and outro music is from The Hug by The Freak Fandango Orchestra / CC BY-SASponsored By:Rudderstack:  RudderStack provides all your customer data pipelines in one platform. You can collect, transform, and route data across your entire stack with its event streaming, ETL, and reverse ETL pipelines. RudderStack’s warehouse-first approach means it does not store sensitive information, and it allows you to leverage your existing data warehouse/data lake infrastructure to build a single source of truth for every team. RudderStack also supports real-time use cases. You can Implement RudderStack SDKs once, then automatically send events to your warehouse and 150+ business tools, and you’ll never have to worry about API changes again. Visit [dataengineeringpodcast.com/rudderstack](https://www.dataengineeringpodcast.com/rudderstack) to sign up for free today, and snag a free T-Shirt just for being a Data Engineering Podcast listener.Upsolver: 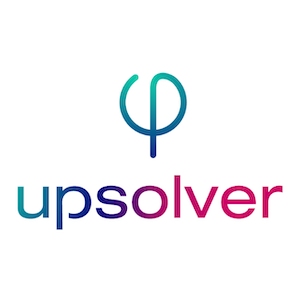 Build Real-Time Pipelines. Not Endless DAGs! Creating real-time ETL pipelines is extremely time-consuming and engineering intensive. Why? Because when we attempt to shoehorn a 30-year old batch process into a real-time pipeline, we create an orchestration hell that makes every pipeline a data engineering project. Every pipeline is composed of transformation logic (the what) and orchestration (the how). If you run daily batches, orchestration is simple and there’s plenty of time to recover from failures. However, real-time pipelines with per-hour or per-minute batches make orchestration intricate and data engineers find themselves burdened with building Direct Acyclic Graphs (DAGs), in tools like Apache Airflow, with 10s to 100s of steps intended to address all success and failure modes, task dependencies and maintain temporary data copies. Ori Rafael, CEO and co-founder of Upsolver, will unpack this problem that bottlenecks real-time analytics delivery, and describe a new approach that completely eliminates the need for orchestration, so you can remove Airflow from your development critical path and deliver reliable production pipelines quickly. Go to [dataengineeringpodcast.com/upsolver](dataengineeringpodcast.com/upsolver) to start your 30 day trial with unlimited data, and see for yourself how to avoid DAG hell.Datafold: 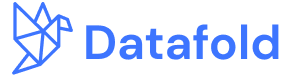 Datafold helps you deal with data quality in your pull request. It provides automated regression testing throughout your schema and pipelines so you can address quality issues before they affect production. No more shipping and praying, you can now know exactly what will change in your database ahead of time. Datafold integrates with all major data warehouses as well as frameworks such as Airflow & dbt and seamlessly plugs into CI, so in a few minutes you can get from 0 to automated testing of your analytical code. Visit our site at [dataengineeringpodcast.com/datafold](https://www.dataengineeringpodcast.com/datafold) today to book a demo with Datafold.Linode: 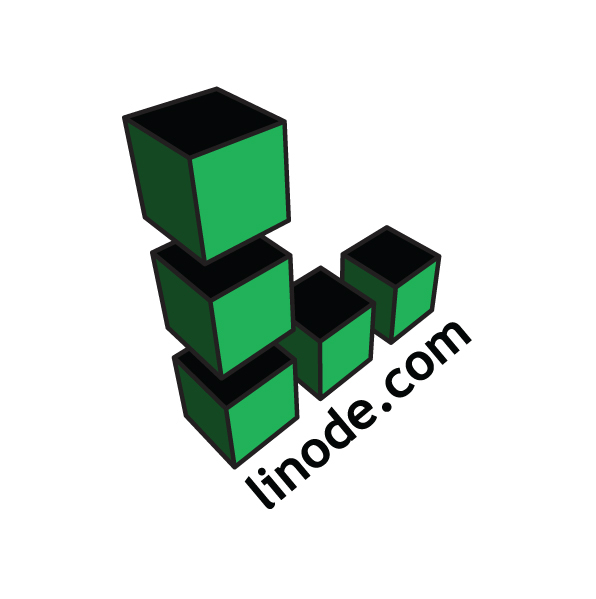 Your data platform needs to be scalable, fault tolerant, and performant, which means that you need the same from your cloud provider. Linode has been powering production systems for over 17 years, and now they’ve launched a fully managed Kubernetes platform. With the combined power of the Kubernetes engine for flexible and scalable deployments, and features like dedicated CPU instances, GPU instances, and object storage you’ve got everything you need to build a bulletproof data pipeline. If you go to: [dataengineeringpodcast.com/linode](https://www.dataengineeringpodcast.com/linode) today you’ll even get a $100 credit to use on building your own cluster, or object storage, or reliable backups, or… And while you’re there don’t forget to thank them for being a long-time supporter of the Data Engineering Podcast!Support Data Engineering Podcast