#21 Gaussian Processes, Bayesian Neural Nets & SIR Models, with Elizaveta Semenova
Learning Bayesian Statistics - Podcast autorstwa Alexandre Andorra - Środy
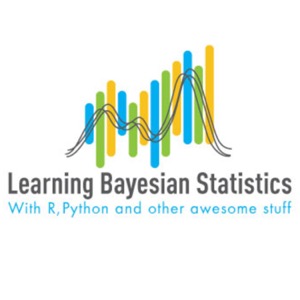
Kategorie:
I bet you heard a lot about epidemiological compartmental models such as SIR in the last few months? But what are they exactly? And why are they so useful for epidemiological modeling?
Elizaveta Semenova will tell you why in this episode, by walking us through the case study she recently wrote with the Stan team. She’ll also tell us how she used Gaussian Processes on spatio-temporal data, to study the spread of Malaria, or to fit dose-response curves in pharmaceutical tests.
And finally, she’ll tell us how she used Bayesian neural networks for drug toxicity prediction in her latest paper, and how Bayesian neural nets behave compared to classical neural nets. Ow, and you’ll also learn an interesting link between BNNs and Gaussian Processes…
I know: Liza works on _a lot_ of projects! But who is she? Well, she’s a postdoctorate in Bayesian Machine Learning at the pharmaceutical company AstraZeneca, in Cambridge, UK.
Elizaveta did her masters in theoretical mathematics in Moscow, Russia, and then worked in financial services as an actuary in various European countries. She then did a PhD in epidemiology at the University of Basel, Switzerland. This is where she got interested in health applications – be it epidemiology, global health or more small-scale biological questions. But she’ll tell you all that in the episode ;)
Our theme music is « Good Bayesian », by Baba Brinkman (feat MC Lars and Mega Ran). Check out his awesome work at https://bababrinkman.com/ !
Links from the show:
- Liza on Twitter: https://twitter.com/liza_p_semenova
- Liza on GitHub: https://github.com/elizavetasemenova
- Liza's blog: https://elizavetasemenova.github.io/blog/
- A Bayesian neural network for toxicity prediction: https://www.biorxiv.org/content/10.1101/2020.04.28.065532v2
- Bayesian Neural Networks for toxicity prediction -- Video presentation: https://www.youtube.com/watch?v=BCQ2oVlu_tY&t=751s
- Bayesian workflow for disease transmission modeling in Stan: https://mc-stan.org/users/documentation/case-studies/boarding_school_case_study.html
- Andrew Gelman's comments on the SIR case-study: https://statmodeling.stat.columbia.edu/2020/06/02/this-ones-important-bayesian-workflow-for-disease-transmission-modeling-in-stan/
- Determining organ weight toxicity with Bayesian causal models: https://www.biorxiv.org/content/10.1101/754853v1
- Material for Applied Machine Learning Days ("Embracing uncertainty"): https://github.com/elizavetasemenova/EmbracingUncertainty
- Predicting Drug-Induced Liver Injury with Bayesian Machine Learning: https://pubs.acs.org/doi/abs/10.1021/acs.chemrestox.9b00264
- Ordered Logistic Regression in Stan, PyMC3 and Turing: https://medium.com/@liza_p_semenova/ordered-logistic-regression-and-probabilistic-programming-502d8235ad3f
- PyMCon website: https://pymc-devs.github.io/pymcon/
- PyMCon Call For Proposal: https://pymc-devs.github.io/pymcon/cfp
- PyMCon Sponsorship Form: https://docs.google.com/forms/d/e/1FAIpQLSdRDI1z0U0ZztONOFiZt2VdsBIZtAWB4JAUA415Iw8RYqNbXQ/viewform
- PyMCon Volunteer Form: https://docs.google.com/forms/d/e/1FAIpQLScCLW5RkNtBz1u376xwelSsNpyWImFisSMjZGP35fYi2QHHXw/viewform