117. Beena Ammanath - Defining trustworthy AI
Towards Data Science - Podcast autorstwa The TDS team
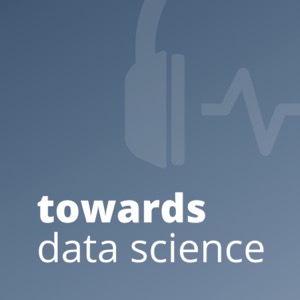
Kategorie:
Trustworthy AI is one of today’s most popular buzzwords. But although everyone seems to agree that we want AI to be trustworthy, definitions of trustworthiness are often fuzzy or inadequate. Maybe that shouldn’t be surprising: it’s hard to come up with a single set of standards that add up to “trustworthiness”, and that apply just as well to a Netflix movie recommendation as a self-driving car. So maybe trustworthy AI needs to be thought of in a more nuanced way — one that reflects the intricacies of individual AI use cases. If that’s true, then new questions come up: who gets to define trustworthiness, and who bears responsibility when a lack of trustworthiness leads to harms like AI accidents, or undesired biases? Through that lens, trustworthiness becomes a problem not just for algorithms, but for organizations. And that’s exactly the case that Beena Ammanath makes in her upcoming book, Trustworthy AI, which explores AI trustworthiness from a practical perspective, looking at what concrete steps companies can take to make their in-house AI work safer, better and more reliable. Beena joined me to talk about defining trustworthiness, explainability and robustness in AI, as well as the future of AI regulation and self-regulation on this episode of the TDS podcast. Intro music: - Artist: Ron Gelinas - Track Title: Daybreak Chill Blend (original mix) - Link to Track: https://youtu.be/d8Y2sKIgFWc Chapters: 1:55 Background and trustworthy AI 7:30 Incentives to work on capabilities 13:40 Regulation at the level of application domain 16:45 Bridging the gap 23:30 Level of cognition offloaded to the AI 25:45 What is trustworthy AI? 34:00 Examples of robustness failures 36:45 Team diversity 40:15 Smaller companies 43:00 Application of best practices 46:30 Wrap-up